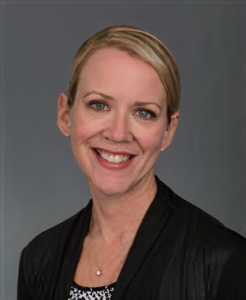
Author: Laurie A. Henneborn, Managing Director, Accenture Research at Accenture
Imagine finding your dream job only to be rejected because a computer algorithm misinterpreted your facial expressions or decided that the tone of your voice during your initial interview made you a less-than-desirable candidate.
Approximately 76% of organizations with 100 or more employees use algorithms to assess performance on hiring tests, and 40% use artificial intelligence (AI) when screening potential candidates. But while the vast majority of C-suite executives believe AI is the key to growth, many are unaware that the data used to select qualified employees may not include critical considerations of disability, including physical, cognitive, sensory, visible and invisible.
This has coincided with a disproportionately low representation in the workforce among people with disabilities. Although one in four US adults and more than one billion globally live with some form of disability – encompassing every age, ethnicity, gender identity, race, sexual orientation and socioeconomic status – 50% of those in the US are more likely to be unemployed than their nondisabled working age counterparts.
When designed, developed, and used ethically and responsibly, AI has the power to change the game for employers and employees. It has the potential to facilitate the employment journey for persons with disabilities, helping organizations identify candidates (and vice versa) and enabling productive engagement at work. And it can drive an inclusive culture of confidence in this underutilized segment of the workforce. The challenge is making sure that the underlying algorithms and data sets powering AI are trustworthy and free of bias.
Closing the Bias Gap
There’s a well-known story about how Amazon trained a resume-screening algorithm by analyzing resumes the company had received over the past ten years. As the company soon found out, there was just one problem: because of the larger number of men vs. women who applied for jobs, the algorithm inadvertently learned to penalize resumes that included terms such as “women’s” or reflected a degree from a women’s college.
Amazon corrected the tool, but the incident highlights that existing data sets—which AI uses to form conclusions and power decisions—lack a satisfactory level of diversity and inclusion. What happened in the Amazon example, where the AI developed a bias against women, could happen to people with disabilities as well. For example, AI could use current data sets that underrepresent candidates with disabilities, risking excluding people with disabilities from the talent pool.
To help close the bias gap, organizations can assess algorithms and apply inclusive design principles. This includes designing AI solutions that consider the needs of all users to prevent unintended consequences, such as bias and discrimination.
Incorporating inclusive design may also require a cultural shift within organizations. This often comes down to self-disclosure—many with disabilities successfully conceal conditions such as dyslexia, autoimmune diseases such as multiple sclerosis, or chronic mental health conditions. The lack of disability representation in data sets won’t improve if people aren’t comfortable being open and giving their input. Empowering people to feel comfortable disclosing will require a highly inclusive culture.
R(AI)S Your AI Game
To ensure that AI positively impacts people with disabilities and helps them achieve their goals, we developed the following guiding principles: R (AI)S for Responsible, Accessible, Inclusive, and Secure. We explore these four principles in-depth in Accenture’s new report created in partnership with Disability:IN and the American Association of People with Disabilities (AAPD), AI for Disability Inclusion: Enabling Change with Advanced Technology. Here is the high-level on how they can help to reduce bias against people with disabilities in your organization:
Responsible: Adopt and scale AI responsibly and ethically; innovate with purpose; and place a premium on compliance, accountability and transparency. Sample questions that organizations can ask to assess their starting point include:
- Are we assessing and communicating how our AI systems impact people throughout the employee lifecycle?
- Is an independent team evaluating our system and/or empowered to stop and correct negative impacts?
Accessible: Ensure that all AI endeavors put a premium on accessibility, including the features and functionality of the tool itself, the capabilities of vendors involved, and the experience of the person with disability surrounding its use. Sample questions to get started include:
- Are we engaging employees with disabilities in our accessibility efforts including, but not limited to, usability testing?
- Are we defining accessibility and usability as a requirement in our procurement contracts?
- Are we embedding accessibility into our relevant policies?
Inclusive: Act with fairness in mind with AI; use inclusive design approaches that incorporate the lived experience of persons with disabilities; use de-biasing techniques to create a culture of equality and inclusion. Sample questions organizations can ask themselves include:
- Are we directing and/or incentivizing our talent development teams to use inclusive design principles?
- Are we conducting regular fairness assessments/audits of algorithms (and the underlying data) to understand and act upon any potential negative impacts?
Secure: Ensure that using AI will not put privacy at risk; recognize that individuals should not need to ask for help because of their disability. Sample questions organizations can ask themselves include:
- Do we have an internal governance model in place ensuring safe, secure and fair deployment and operation of AI systems?
- Do we recognize that candidates with disabilities have the same reasonable expectations of privacy as do other candidates?
These are just some ways to ensure that AI is helping and not inadvertently working against people with disabilities. From the hiring process to life at work and employee evaluations, it’s critical to assess the potential consequences of AI deployment on every part of the employment experience and re-think algorithms and assumptions to ensure a fair and inclusive work environment.